RAdaBuff
Facts
Academic Partner: The School of Engineering and Jönköping International Business School at Jönköping University
Industrial Partners: BUFAB External link, opens in new window., Fagerhult
External link, opens in new window., GKN Aerospace
External link, opens in new window., Siemens Energy
External link, opens in new window., Tranter
Project duration: 2021-2024
Research team:
Joakim Wikner (Project leader) External link, opens in new window.
Helena Forslund External link, opens in new window.
Lisa Hedvall External link, opens in new window.
Stig-Arne Matsson
Cecilia Sönströd External link, opens in new window.
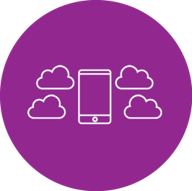
Predicting and dimensioning the need for buffers in the supply chain is a major challenge for many companies. In RAdaBuff we investigate how responsive dimensioning of safety buffers scan be done with the help of data-intensive decision support. The research is carried out in close cooperation with leading manufacturing companies.
Introduction
RAdaBuff (Responsiveness through Adaptive Buffering) is part of the research profile AFAIR focusing on applied AI in industrial organizations. RAdaBuff is part of the research area ”Data-driven development of products and services” (ARA1). It is one of four projects combining data science, logistics and supply chain management named the ”Ontime”-projects”. In the project, we want to contribute to a deeper understanding of how companies can use buffers to reduce and absorb variations in the supply chain and thus maintain a competitive and responsive delivery performance.
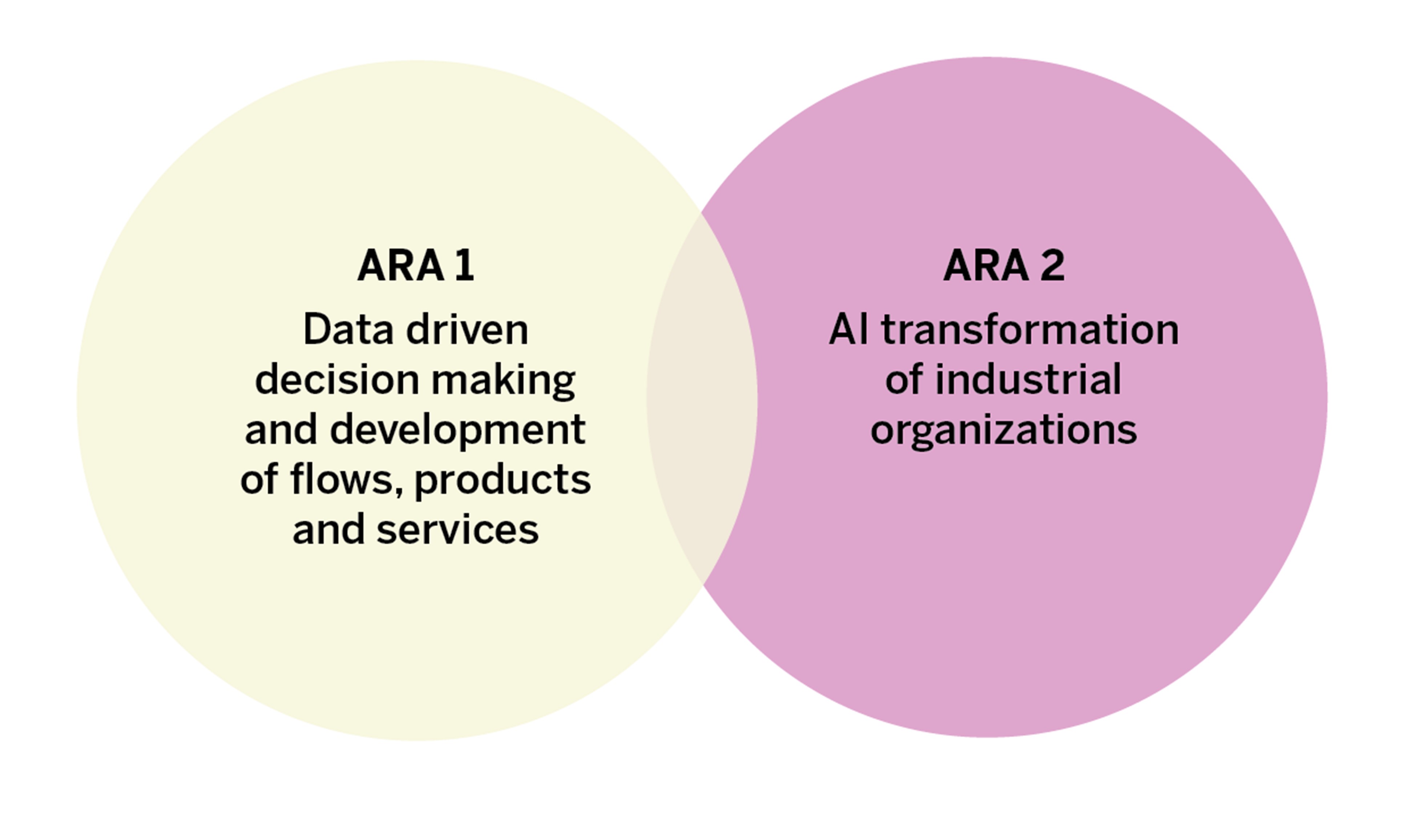
Motivation and purpose
Buffer management is a very important part of the company's supply chain, its leadership and operations. Well-functioning buffers are essential for responsive delivery performance and cost control. The most obvious type of buffer is materials, such as in safety stocks, but also lead-time extensions and temporarily idle resources may represent buffers in lead-times and capacity. By having a proactive approach, companies can gain better control over their delivery capability, but it can also improve cost control regarding materials and time. For example: instead of working overtime, you can use your ”time” buffer. In close cooperation with our industrial partners, we actively work with experiments and measurement of data to understand the function of the buffers, identify which buffers are required and how they can be combined, and to understand why variations occur and how they can be reduced.
Expected results
Our goal is to develop a work process for adoptive control of buffers, consulting of decision support and tools in order to enable responsiveness. This process should contain support linked to each step – and thereby contribute to ensuring the delivery capacity of businesses. We also strive to develop a tool where we work with data-driven decision support that creates the conditions for the use of AI. We are dealing with complex relationships, which is why we have chosen to work in two parallel research projects. While RAdaBuff focuses on the work process, the sister project PredMod focuses on machine learning and prediction.
For more information, contact:
- Professor Logistics
- School of Engineering
- joakim.wikner@ju.se
- +46 36-10 1637
Are you interested in a future collaboration?
- External Relations Manager
- School of Engineering
- linda.bergqvist@ju.se
- +46 36-10 1074