Finding actionable patterns in eye movement and weapon handling within rifle marksmanship
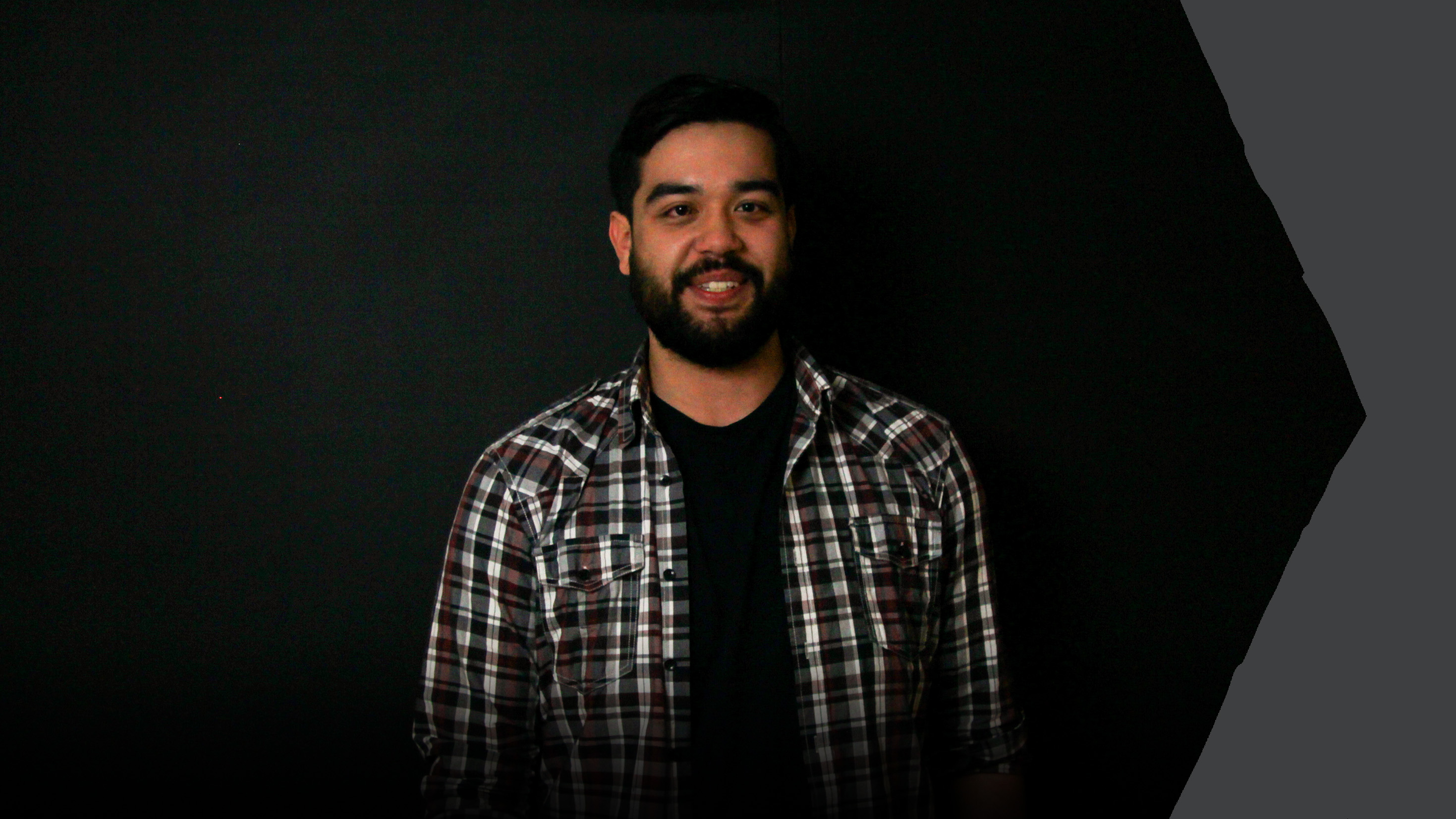
AI and machine learning is well suited to analyze and make predictions on real-world applications, such as rifle marksmanship. In this thesis, a machine learning model is applied on a rifle shooting exercise to give predictions on marksmanship skill.
Rifle marksmanship is a domain where hand-eye coordination plays an important role. Predicting the skill of a shooter based on data from sensors that measure hand-eye coordination is a difficult task which produces complex data. AI and machine learning is a suitable tool to use when trying to create automatic predictions from complex real-world data and can be applied on rifle marksmanship.
How rifle handling and stability affect shooting result may seem intuitive, but it is complicated for a dynamic rifle shooting exercise with multiple targets and where time, and shot placement, play a factor in score. It is even less intuitive how rifle handling and eye movement interact to affect shooting results. Studies have shown that there are differences in eye movement between novice and experienced shooters. Resistance to distractions, ability to fixate on a target, and shooter focus can all be deduced from eye movement.
In collaboration with Saab AB, Training and Simulation, an experiment was conducted using 13 participants of varying levels of marksmanship experience. The participants performed a shooting exercise with multiple targets and with time pressure. During the exercise, weapon and eye movement was measured to create a machine learning model for prediction of shooting results. Eye movement was measured using a wearable eye tracker. A sensor platform consisting of custom created software and hardware is used to measure weapon movement and to synchronize data from multiple sensors. The goal is to produce a machine learning model to help a shooter improve their marksmanship training.