AATrackT: A deep learning network using attentions for tracking fast-moving and tiny objects
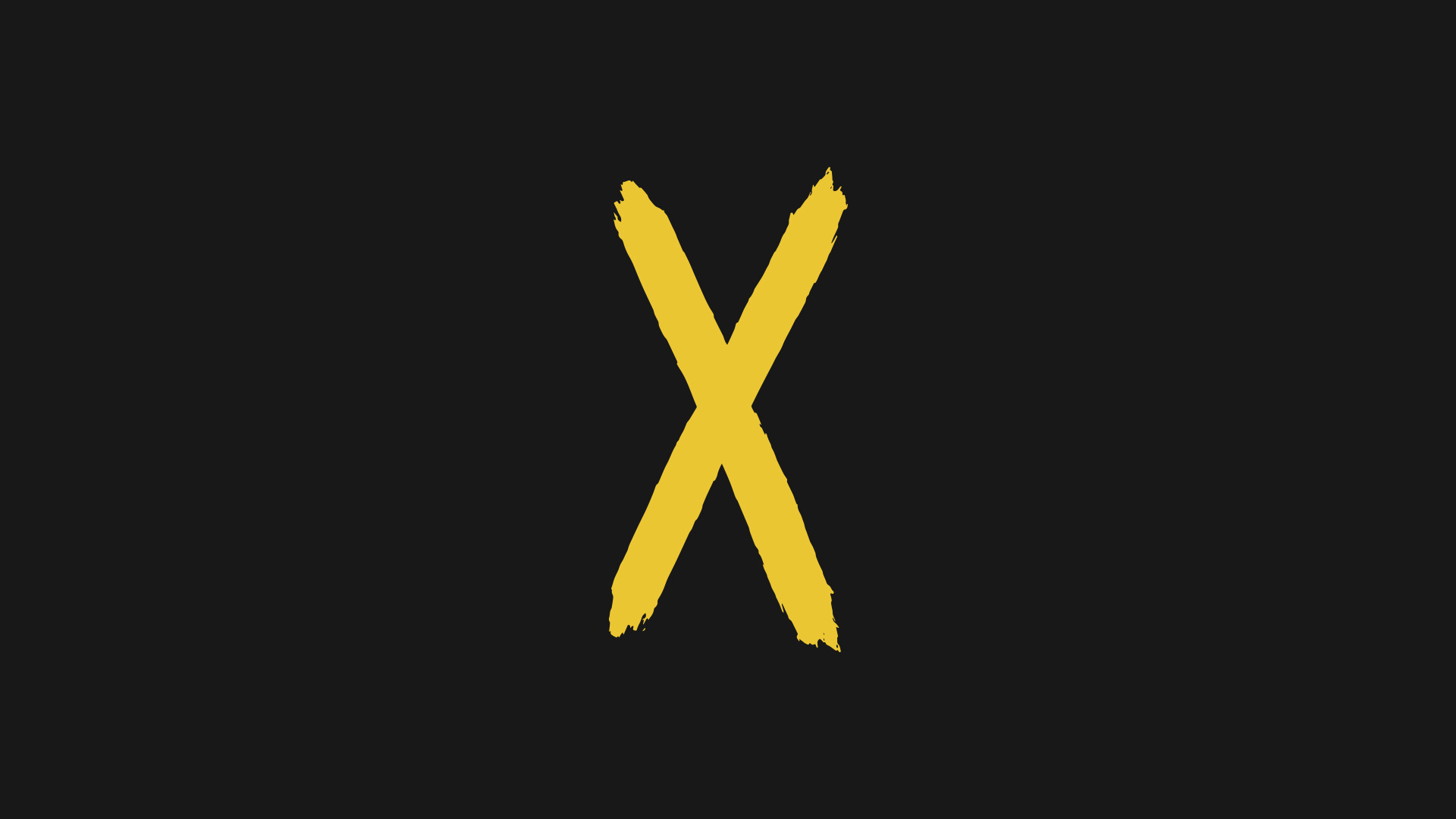
Fredric Lundberg
LinkedIn Länk till annan webbplats, öppnas i nytt fönster.
The objective of this work is to track tiny fast-moving objects e.g., Tennis or Padel balls. By fulfilling this objective, many other features can be implemented consequently. For example, automatically count points, or to provide sport-analysis for different practitioners.
The recent advances in deep learning have made it possible to visually track objects from a video sequence accurately. Moreover, as transformers got introduced in computer vision, new state-of-the-art performances were achieved in visual tracking. However, most of these transformer-based studies have used attentions to correlate the distinguishing factors between target-object and candidate-objects to localise the object throughout the video sequence. These approaches are often not applicable to track extreme small objects, or objects that are moving fast because of the lack of sharp textures. Therefore, the purpose of this study is to improve current methods to track tiny fast-moving objects, with the help of attentions. A deep neural network, named AATrackT, is built to address this gap by referring to it as a visual image segmentation problem. The proposed method is using data extracted from broadcasting videos of the sport Tennis. Moreover, to capture the global context of images, attention augmented convolutions are used as a substitute to the conventional convolution operation. Contrary to what the authors assumed, the experiment showed an indication that using attention augmented convolutions did not contribute to increasing the tracking performance. The main reason is that the spatial resolution of the activation maps of 72x128 is too large for the attention weights to converge. A fair comparison evaluation was conducted between AATrackT and its related work's model TrackNet. Our findings showed that the proposed model performed worse, in relation to its ancestry, with a precision of 0.92, recall of 0.51 and F1-measure of 0.66.