HRV as a predictor of shooting performance
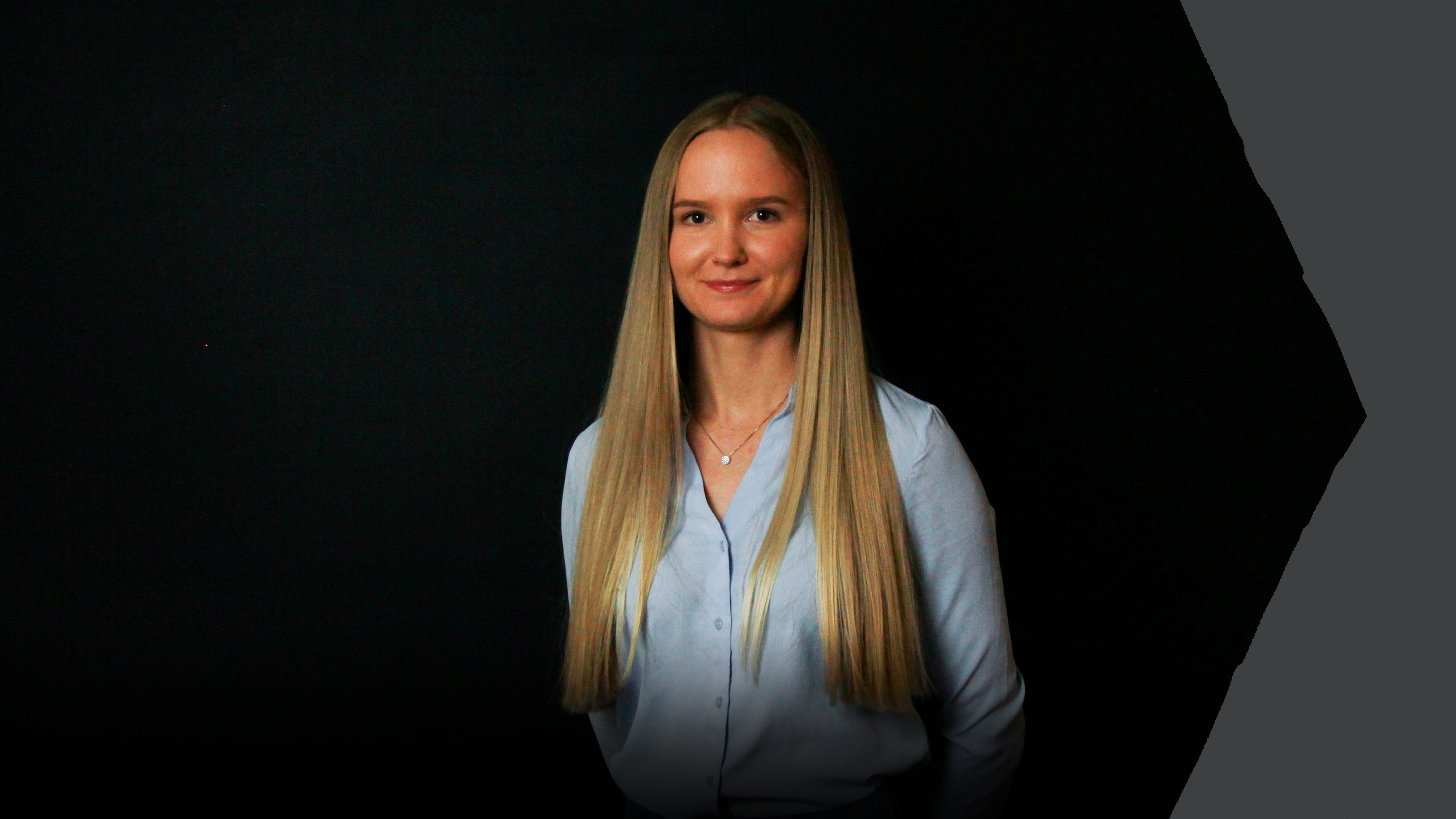
AI and machine learning is increasingly used to gain insights from personal physiological sensor data for the purpose of improving athletic performance. How can measures of cognitive and physical function of a shooter be modeled to aid training and performance?
By modeling measurements of an athlete’s functional state and subsequent performance, an intelligent system may guide individual training and help maximize performance. Heart rate variability (HRV) is a measure of the variation in time between successive heartbeats and indicates the state of the autonomic nervous system. HRV is a marker for health, fitness, recovery, and cognitive function and can be measured using pulse sensors. There is supporting evidence across tasks and populations that higher HRV is associated with increased performance in tasks that require active attention and working memory.
Target shooting is a complex, fine motor task in both sports and military. While cognitive and physical function measured by HRV immediately prior to and during shooting is correlated with performance, it remains unclear whether daily changes in HRV measured during sleep is correlated with shooting performance over several weeks or months.
To explore this problem, an experiment was carried out in collaboration with Saab AB, Training and Simulation. Daily HRV and simulator rifle shooting performance was collected from ten experienced shooters during five weeks. In addition to experiment design and execution, processing of data and analysis using machine learning was done. By using machine learning, the objective is to predict shooting performance based on the functional state of the shooter a certain day. The ultimate goal is to provide shooters with actionable information to improve training and performance.